Sophon MLOps
AI Capability Operations Platform
Distributed Algorithms
/
Visual Modelling
/
Code Modelling
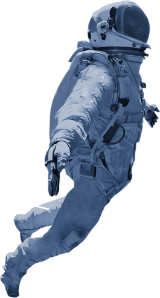
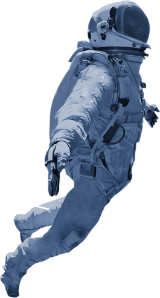
Product Introduction
Sophon MLOps is an enterprise-class AI capability operation platform built on cloud-native architecture, which provides enterprise customers with easy-to-use, efficient, secure and reliable AI capability operation services throughout the lifecycle of machine learning models through unified management, unified operation and maintenance, unified application and unified monitoring.It can help customers manage the ever-growing machine learning models at scale, improve model usage efficiency, reduce model management costs, and control the risk of model production environments.
What an Sophon MLOps offer?
Service Management
It provides the comprehensive management of model services including monitoring, testing, auditing and evaluation. Specifically, it supports real-time monitoring of the running status of model services, rapid testing and verification of service availability, independent view of the full monitoring indicators of each service, and view of the performance evaluation report of model services, which providies a reference for model iteration.
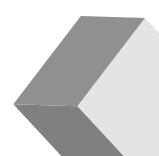
Service Reasoning
It provides a visual operation interface and can quickly build model service reasoning diagrams supporting XGBoost, MLflow, TensorFlow, PyTorch, Scikit-learn, Spark-mllib and other algorithm frameworks. It also supports custom configuration of complex inference logic containing multiple models, significantly saving model configuration costs.
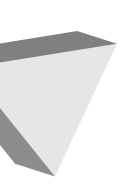
Service Releasing
It can provide zero-code deployment of model services and support customized configuration of elastic scaling strategy, multi-version traffic allocation strategy, as well as on-demand allocation of CPU, GPU, and memory resources for each node of the inference graph, so as to easily realize multi-instance load balancing, grayscale release and A/B testing of model services.
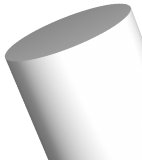
Model Management
It helps users to unify and manage multi-sources heterogeneous model files, and enables unified deployment of large-scale machine learning models through standardized integration management, significantly reducing model management costs.
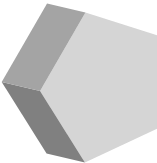
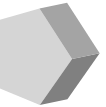
Service Image
It can seamlessly integrate model images in Sophon Base and support rapid deployment of trained machine learning models in Base using MLOps. At the same time, model container images generated by other training platforms can be upload in MLOps for unified management, operation and monitoring.
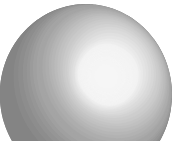
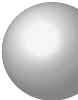
Why should you choose Sophon MLOps?
Integrated Management at Scale
Uniformly manage multi-sources heterogeneous model files and enable uniform deployment of large-scale machine learning models through standardised integration management, thus helping users to significantly reduce model management costs.
Efficient Model Reasoning Services
Help you to quickly build service inference diagrams with graphical interaction, and support you custom configuration of complex reasoning logic containing multiple models, and rapid deployment of visualized model applications, simplifying the complex process of model configuration and deployment.
Multi-dimensional Monitoring and Evaluation
Help you control the risk of the model production environment by monitoring the running status of the model service in real-time. And provide important reference for you to optimize the model in subsequent iterations through multi-dimensional evaluation of the model prediction effect.
Adequate Protection to Privacy and Security
Provide various user rights control to meet the needs of different teams or user roles to restrict the cluster environment, resources and system functions; Guarantee the information isolation of private data among users; And support the approval process for model deployment to control the utilization of service resources.
Application Cases
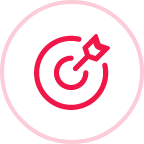
Customer needs
○ In order to meet regulatory compliance requirements and improve the efficiency of model management in the head office and branches, the whole life cycle of models needs to be managed in a unified manner
○ A large number of AI models from different algorithm frameworks have been accumulated in the bank, but they are mostly scattered in many different business departments
○ The lack of a unified process for model deployment in each department and the need to configure a large number of parameters lead to long model deployment cycles, high configuration costs and reduced the development efficiency and the application agility of models.
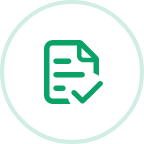
Solutions
○ Use Sophon MLOps to build a bank-wide unified AI model management platform to quickly access the heterogeneous models accumulated in the bank
○ Use MLOps to build a standardized process for model application, the unified construction of model reasoning logic, and the realization of model rapid deployment
○ Continuously monitor all released model services in MLOps to achieve unified operation and maintenance, and evaluate model prediction performance to ensure the accuracy of model predications and stability of model operations
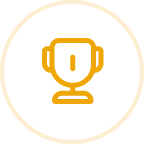
Project results
Successfully solved the problem of managing heterogeneous models by integrating hundreds of machine learning models from multiple algorithmic frameworks across the bank
Significantly reduced the average deployment time and model configuration cost in the process of model applications
Sucessfully supported hundreds of model prediction services online at the same time, and achieved millisecond response for a single piece of data
Significantly shortened the average model iteration cycle by succeeding in going through the full model lifecycle process.
Transwarp, Shaping the Future Data World